
AI in Footwear Merchandising: Advancing Flexibility and Profitability

Footwear merchandisers face challenges unique to the retail space, with extensive colorway and size variance, non-traditional seasons, and long production cycles. Rule of thumb approaches help merchandisers manage this complexity, but often at the cost of financial performance. Explore how artificial intelligence (AI) can augment traditional footwear merchandising approaches, offering unprecedented levels of precision and profitability.
The Multifaceted Challenges of Footwear Merchandising
Although footwear presents challenges at every step of the merchandising process, there are five key areas that are worth additional investigation.
1) Planning: Balancing Creativity with Data-Driven Decisions
The planning phase in footwear merchandising requires a delicate balance between creative intuition and data-driven decision-making. Merchandisers must navigate the intricacies of seasonal transitions, which in the footwear industry are particularly pronounced. Unlike apparel, where layering can extend the wearability of items across seasons, footwear tends to have more distinct seasonal boundaries. This necessitates a nuanced understanding of not just when seasons change, but how consumer behavior shifts in anticipation of these changes.
The challenge of SKU proliferation in footwear is particularly acute. A single style can easily multiply into dozens of SKUs when accounting for size ranges (which can span from children's sizes to extended adult sizes), width options, and colorways. This proliferation not only complicates inventory management but also impacts the entire supply chain, from production to distribution.
💡FOR CONSIDERATION
Some planning activities, like accounting for predictable seasonality, are “human manageable” — but more complex analyses may only be “machine manageable.” SKU proliferation is a great example of the big data problem that challenges modern merchandising teams.
2) Demand Forecasting: Navigating Uncertainty in Style and Fit
Demand forecasting in the footwear industry is fraught with variables that can significantly impact accuracy. Beyond the usual considerations of fashion trends and consumer preferences, footwear merchandisers must contend with the added complexity of fit. A style that sells well in one region may underperform in another due to differences in foot shapes and size preferences among populations.
The impact of external factors on footwear demand can be more pronounced and nuanced than in other fashion categories. For instance, while a rainy season might generally increase demand for waterproof footwear, the specific styles that gain traction can vary widely based on local fashion sensibilities and functional needs.
💡FOR CONSIDERATION
Statistical forecasting models are an effective tool but limited in their ability to incorporate multiple variables and manage high variance. Machine learning models for forecasting are a modern alternative capable of meeting footwear merchandisers’ needs.
3) Buying: Long Lead Times and Minimum Order Quantities
The buying process in footwear is characterized by extended lead times, often ranging from 6 to 9 months from design to delivery. This timeline is further complicated by the industry's reliance on specialized manufacturing processes and materials. The technical requirements for producing a high-quality, comfortable shoe often necessitate partnerships with specialized factories, many of which impose significant minimum order quantities (MOQs).
These MOQs present a particular challenge for footwear brands looking to test new styles or cater to niche markets. The financial commitment required for a full production run can be substantial, putting pressure on merchandisers to make highly accurate buying decisions months in advance of the selling season.
💡FOR CONSIDERATION
Nearshoring and on-demand production methods are often touted as lead time cure-alls, but for merchandising teams lacking supply chain ownership, systemic change like this is unfeasible. Focusing on ownable variables, such as implementing attribute-based demand modeling, may deliver faster impact.
4) Allocation: The Complexity of Size Curves and Channel-Specific Demands
Allocation in footwear merchandising is a multidimensional challenge that goes beyond simply distributing inventory to different locations. Merchandisers must grapple with size curves that can vary significantly across different styles, brands, and even colorways within the same style. The risk of stockouts in popular sizes must be balanced against the potential for overstock in less common sizes.
Additionally, the rise of omnichannel retail has added layers of complexity to the allocation process. Footwear brands must now consider not only the differing demands of brick-and-mortar locations but also the specific requirements of e-commerce fulfillment, which often necessitates a more centralized inventory approach to efficiently handle returns and exchanges due to sizing issues.
💡FOR CONSIDERATION
So-called "inventory-less" retail models offer a compelling solution, but there isn’t a one-size-fits-all approach. Embrace the principle of minimizing stock liability while maximizing choice, but think pragmatically about how you might employ it. Rapid rebalancing based on real-time demand signals, for example, may be a compelling starting point before taking the full inventory-less plunge.
5) Data Analysis: Extracting Actionable Insights from Diverse Data Streams
The challenge in data analysis for footwear merchandising lies not in a lack of data, but in the complexity of synthesizing insights from diverse and often disconnected data streams. Point-of-sale data, e-commerce analytics, social media trends, and competitive intelligence all play crucial roles in informing merchandising decisions.
However, the true difficulty lies in integrating these data sources to create a coherent picture of market demand and consumer behavior. Traditional data analysis methods often struggle to handle the volume and variety of data available, leading to delays in insight generation that can be costly in a fast-moving industry.
💡FOR CONSIDERATION
Tackling big data analysis problems may be less about desire and more about process. Evaluate whether your people, organization, or culture are impacting your ability to leverage data appropriately.
AI Solutions: Precision Tools for Complex Challenges
Artificial intelligence offers a suite of capabilities uniquely suited to address the complex challenges faced by footwear merchandisers. By leveraging advanced algorithms and machine learning models, AI can provide granular levels of insight and reduced manual workloads across the merchandising process.
Advanced Demand Forecasting
AI-powered demand forecasting models can integrate a vast array of variables, from historical sales data and social media trends to weather patterns and economic indicators. These models can identify subtle correlations and patterns that might escape human analysis, leading to more accurate predictions of demand across different styles, sizes, and regions.
For example, an AI system might detect that a particular boot style tends to see increased demand not just in cold weather, but specifically in urban areas with robust public transportation systems. This level of granularity in forecasting can significantly improve inventory planning and reduce the risk of overstock or stockouts.
Dynamic Inventory Optimization
AI can continuously analyze sales data, inventory levels, and incoming orders to optimize inventory distribution across channels and locations in real-time. This dynamic approach to inventory management can help footwear brands maintain optimal stock levels, reducing carrying costs while minimizing lost sales due to stockouts.
And AI systems can learn from past performance to improve size curve predictions, ensuring that the right sizes are available in the right locations. This is particularly valuable in managing the complex size distributions typical in footwear retail.
Predictive Trend Analysis
By analyzing vast amounts of data from social media, fashion blogs, and online marketplaces, AI can identify emerging style trends far earlier than traditional methods. This predictive capability can inform design and buying decisions, allowing footwear brands to stay ahead of market trends and adjust their offerings accordingly.
Stepping into a Data-Driven Footwear Future
The integration of AI into footwear merchandising represents a significant leap forward in addressing the industry's unique challenges. By providing deeper insights, more accurate forecasts, and automated optimization across the merchandising process, AI empowers merchandisers to make more informed decisions and operate with greater efficiency.
As the footwear industry continues to evolve, embracing these AI-driven solutions will be crucial for brands looking to maintain a competitive edge. The future of footwear merchandising lies in the seamless integration of human expertise and AI capabilities, creating a synergy that can navigate the complexities of the market with unprecedented precision.
Learn more about how AI can revolutionize your merchandise planning strategies and footwear allocation processes. Take the first step towards a more data-driven, efficient, and profitable future in footwear merchandising.
Recommended resources
See all resourcesRecommended resources
See all resourcesMake Forecasting Your
Superpower
See how AI tailored to your unique business can deliver
insights that boost margin.
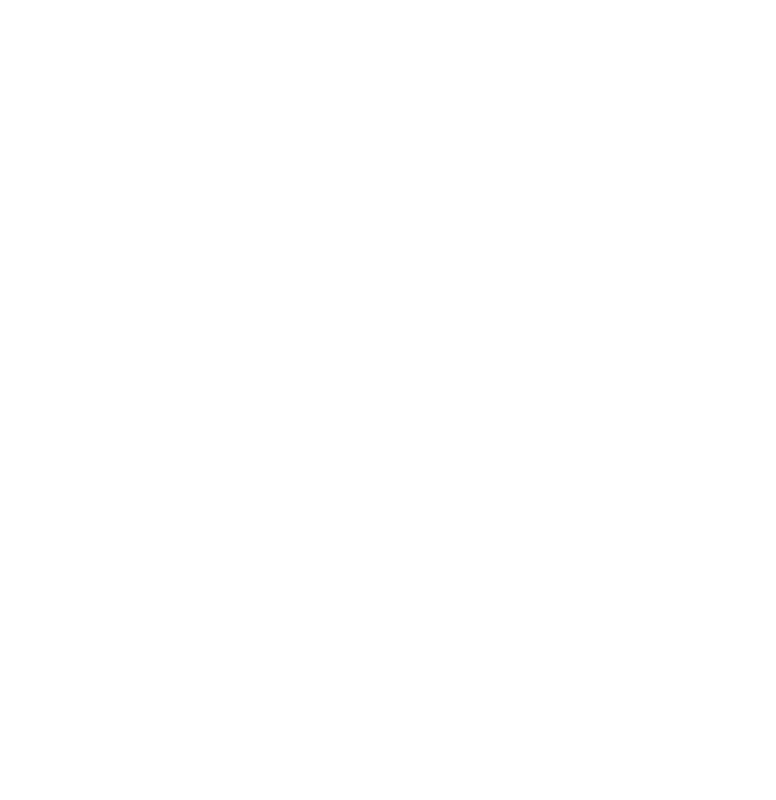