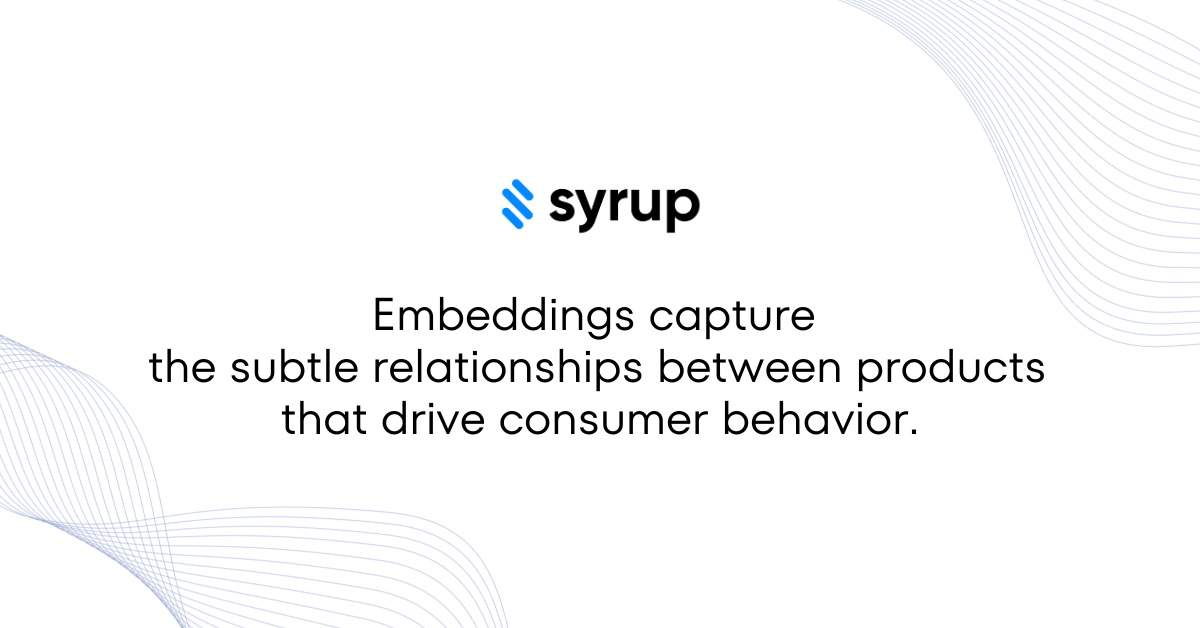
Why Embeddings are Essential for Newness Demand Planning
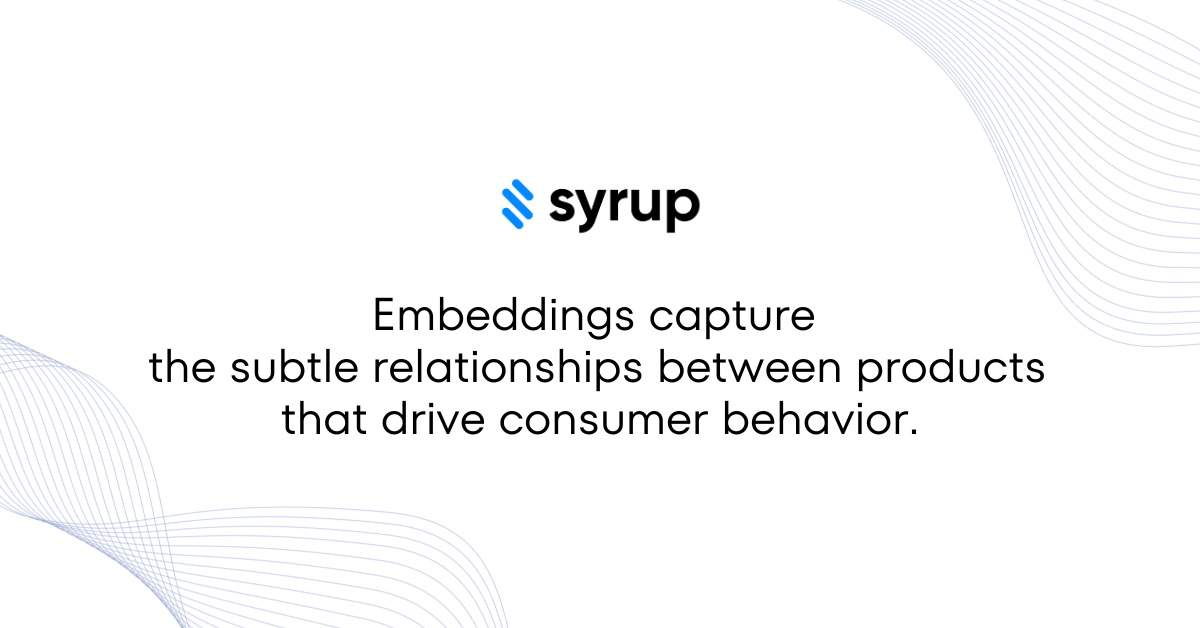
Traditional demand forecasting methods are no longer sufficient for apparel/footwear retailers with a heavy focus on newness. Embeddings, a powerful AI technology, are the ideal tool to solve this challenge.
Embedding-based models demonstrate significant improvements in forecast accuracy compared to traditional methods, particularly for new products. This translates directly to reduced markdowns, better in-stock positions, and improved financial performance.
But having capacity to put embeddings into place requires technical expertise, compute power, and resourcing that many brands simply don’t have access to. And that’s where Syrup comes in…but more on that later.
The Unique Challenge of Newness Forecasting in Apparel/Footwear
Apparel/footwear merchandising teams face a fundamental forecasting challenge: predicting demand for products without sales history. Traditional forecasting methods rely on historical data, but this approach fails when dealing with new products, which typically constitute 40-60% of an assortment each season.
The mechanics of newness forecasting are important for multiple use cases. You might need to generate a newness allocation forecast (you’ve bought it and sold it in Store A, but you’ve never sold it in a Store B) in which case you have no prior sales history of the product in that store, but may have prior sales history from other stores to build upon.
Or you may need a newness buying forecast, (you’ve never sold it period, but you still need to know how much to buy) which lacks even that comparable sales history. Most likely the inclination at times is to just guess — but there are better ways to go about solving this.
The stakes are high. Overestimating demand leads to excess inventory requiring markdowns, eroding margins and sustainability goals. Underestimating means missed sales opportunities and disappointed customers. The industry needs a better way to forecast demand for new products by capturing the subtle relationships between products that drive consumer behavior.
Understanding Embeddings: Product DNA in Vector Space
Embeddings are mathematical representations that capture a product's essential characteristics in high-dimensional vector space. These dense numerical vectors encode complex product attributes in a format that machine learning models can process efficiently.
Text embeddings capture semantic meaning from product descriptions, technical specifications, and marketing copy. They understand that a "midi dress with ruffle detail" shares more characteristics with a "knee-length dress with feminine flourishes" than with a "maxi dress with clean lines," even when traditional keyword matching would miss these nuances.
Image embeddings analyze product photos to understand visual similarities that words alone can't capture. They recognize similar silhouettes, patterns, and design elements across different products, providing a mathematical representation of visual merchandising expertise. As an added bonus, they can also capture more nuanced attributes than text embeddings can — picking up on a “style” or “vibe” that may be difficult to translate into words.
How Embeddings Enable Advanced Machine Learning for Retail
Models can process embedding vectors to understand complex relationships between products and identify patterns that drive demand. By incorporating both text and image embeddings, models can capture a comprehensive view of product similarity that combines visual, descriptive, and functional characteristics.
Embeddings are used in two key ways. For one, they can serve as inputs for machine learning models, particularly deep neural networks and transformer architectures — pre-formulated embeddings are provided to the model.
In addition, embeddings can be learned by the Neural Network. The network picks up on the (dis)similarities between products that allow it to best optimize its objective, which is typically accurately predicting future sales. These learned embeddings are encoded so that when the model sees a new product, it can extract the attributes from previous observations and apply learnings from them to the new product.
Excitingly, the model will automatically optimize which set of embeddings is most important to achieve the highest accuracy. No subjective interpretations required.
In sum, the network is not only learning what makes products similar in terms of attributes or demand profiles, it is also learning whether a given product is a substitute for or complement to other products.
Merchandising Use Cases for Embeddings
Whether forecasting for a new item that has never hit shelves, fine-tuning allocation strategies in the face of changing demand, or anything in between, embeddings offer merchandising teams access to a wealth of impactful workflow improvements.
Assortment Strategy
A key selling point of embeddings is its ability to understand the root causes for cannibalization or halo effect for a product in relation to other products in the assortment. Understanding product relationships through embeddings helps optimize category mix and identify gaps or redundancies in product offerings.
Pre-season Planning
Embeddings enable accurate forecasting for new products by identifying truly comparable items from previous seasons, improving initial buy decisions and allocation strategies.
In-season Management
When early sales signals emerge or new trends catch fire, embedding-based models can rapidly adjust forecasts across similar items, enabling proactive inventory management.
Implementation Challenges and Technical Requirements for Newness Forecasts
Implementing embeddings effectively requires specific technical capabilities and machine learning expertise. Not all model architectures can effectively utilize embeddings — they're best suited for advanced neural network architectures like transformers, convolutional neural networks (CNNs), and certain types of recurrent neural networks (RNNs).
Traditional statistical models and basic machine learning approaches like random forests or linear regression cannot learn embedding vectors (although in certain cases they can use embeddings as inputs). Even with compatible model architectures, significant expertise is required to:
- Design appropriate embedding dimensions and training approaches
- Handle the computational complexity of embedding-based models
- Integrate embedding signals with other demand drivers
- Validate and interpret model outputs
How Syrup Enables Brands to Improve Newness Forecasting with Embeddings
Syrup has developed sophisticated systems to help apparel/footwear retailers leverage the power of embeddings without building complex technical infrastructure. Our approach addresses key implementation challenges.
First, we maintain high data quality standards through automated cleaning and standardization processes for product data and images. This ensures consistent, reliable embedding generation across diverse product catalogs.
Next, we are flexible — one size fits all is not a good data science strategy. When appropriate, our team leverages best in class open source models to augment our non-NN models. These tools are proven to deliver results, and when combined with our configurable models, we have found great success meeting the needs of our customer base.
But we also have the infrastructure to train our own embeddings. Our Large Demand Sensing Model (LDSM) is a neural network that combines multiple embedding types with traditional demand signals, creating a comprehensive view of product relationships and demand drivers. This sophisticated neural network architecture is specifically designed to train and generate embedding vectors effectively.
Finally, we work closely with merchandising teams to validate embedding-related insights, ensuring our models are capturing meaningful product relationships while maintaining the ability to scale across large assortments.
By partnering with Syrup, retailers can drastically improve their newness forecasting approach. We offer access to state-of-the-art embedding-based forecasting — a system that would be incredibly challenging for any brand to develop internally. And our platform delivers the benefits of advanced AI while integrating seamlessly with existing planning processes. It’s a win-win for merchandising and tech teams hoping to improve business performance.
Recommended resources
See all resourcesRecommended resources
See all resourcesMake Forecasting Your
Superpower
See how AI tailored to your unique business can deliver
insights that boost margin.
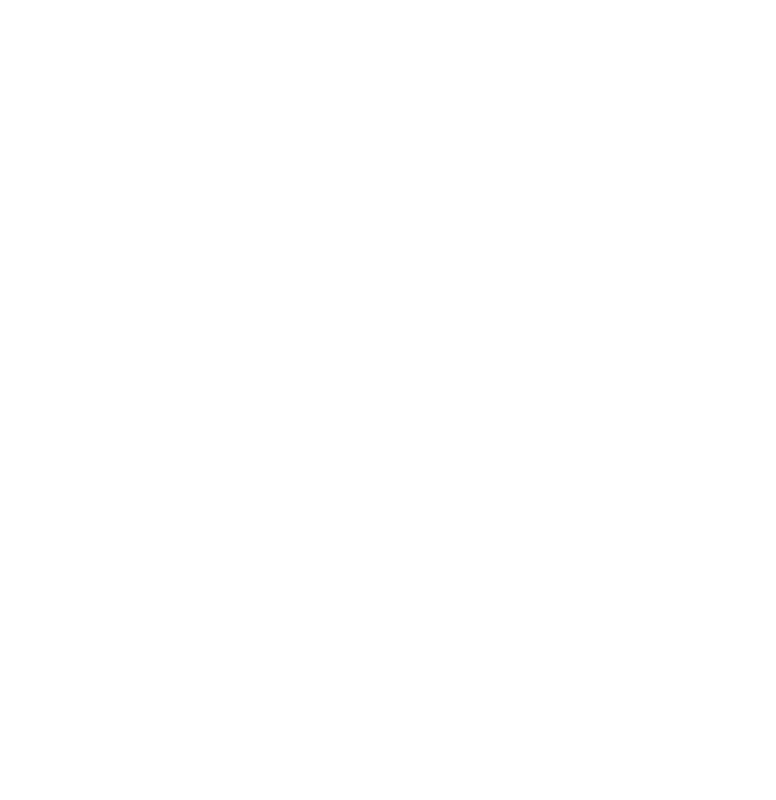