
Production-Scale Demand Forecasting: Reflections On The VN1 Forecasting Competition

The VN1 Forecasting Competition, which ran from September to October 2024, challenged approximately 250 participants to forecast 13 weeks of sales across 15,000 e-vendor SKU combinations. Co-sponsor Nicolas Vandeput's analysis of the competition results demonstrated perfectly the intricate dance between model complexity and performance.
ML models like LGBM and ensemble model approaches achieved impressive accuracy in this competition setting — and yet, a simple baseline approach also managed to score well, finishing 12th overall.
The competition yielded valuable insights about model selection and evaluation frameworks in a discrete, controlled setting. But in everyday merchandising, discrete and controlled are rarely the reality.
For production systems, robust and scalable engineering processes, access to data that can be processed and ingested at scale, and excellent feature engineering end up being more important than the choice of model architectures at the end of the day.
And it’s features like these that make the difference between lab experiments and value-adding products like Syrup’s platform.
The Impact of Missing Inventory Data and Metric Decisions
The competition's dataset structure, while ideal for comparing algorithmic approaches, highlights a significant limitation in demand forecasting without inventory data. Zero sales periods in historical data can represent either genuine lack of demand or stockouts. This systematic bias typically leads to underforecasting, particularly during high-demand periods when stockouts are more likely to occur.
This challenge is not unique to the competition environment — most retailers operate with incomplete or missing inventory data, making this dataset particularly relevant to real-world demand forecasting scenarios.
One key to improving forecast accuracy lies in augmenting these incomplete datasets with complementary data sources. This is where Syrup's data aggregation and unification capabilities provide significant value, incorporating multiple data streams to help minimize the impact of missing inventory data and to provide a more complete picture of true demand patterns.
The competition's chosen metric (MAE% + |Bias%|) effectively evaluates general forecast accuracy but doesn't specifically penalize under-forecasting due to its use of absolute values. In the real world, the asymmetric costs of over- versus under-forecasting often necessitate additional evaluation metrics that account for inventory carrying costs and stockout penalties. There are legitimate business consequences when focusing on only half of the picture.
After all, most brands make conscious strategic decisions that tip the scale toward stockouts or overstocks, and it’s important to carefully manage this tradeoff by ensuring the forecast approaches you choose are aligned with your desired outcome. How you measure plays an oversized role in determining your success — and great strategic partners like Syrup can help you identify and impact the metrics that matter most to your business.
Segmentation and Model Selection
While some competitors implemented segmentation based on seasonality, production forecasting systems benefit from more comprehensive segmentation strategies. Exploratory Data Analysis (EDA) – the systematic process of investigating data patterns and relationships using statistical and visualization techniques – often reveals distinct demand patterns that warrant different modeling approaches:
- High-volume SKUs with stable demand patterns typically benefit from statistical models that can capture subtle trends and seasonality.
- Long-tail SKUs with intermittent demand patterns often perform better with simpler approaches.
- Products with strong seasonal components require specific handling of cyclical patterns and season length variation.
- Highly volatile products with limited sales history and sudden changes in demand may necessitate advanced models like Neural Networks — they can learn the nuanced relationships between features and target, and then extract signal from noise.
This segmentation extends beyond model selection to include differentiated feature engineering strategies and evaluation metrics for each segment. Syrup's ensemble modeling approach leverages these segmentation insights by dynamically combining multiple models, each optimized for specific demand patterns. This architectural design allows us to capture the nuances of different product segments, providing superior accuracy compared to one-size-fits-all solutions.
Production System Architecture
The competition highlighted interesting performance characteristics, particularly regarding inference time. But production forecasting systems face several challenges not present in competition environments.
First, integration of inventory, pricing, and promotional data at scale is a hurdle that many brands still struggle with, and top-tier providers like Syrup make extraordinary efforts to de-silo this important data.
In addition, new product introductions and product discontinuations require constantly updated assortment integration feeds, and likewise, volatile demand patterns require a system that can dynamically adapt.
And finally, brands need their systems to achieve all this with significantly faster processing times than seen in the competition while maintaining comparable accuracy levels.
At Syrup, we've implemented these competition-proven principles at scale through four key tentpoles:
- Parallel processing architectures that efficiently handle millions of SKU-location combinations
- Automated model selection frameworks that dynamically choose appropriate models based on demand patterns
- Robust feature engineering pipelines that incorporate numerous customer data points (inventory, transactions, pricing, product attributes) and external data points where they add value (e.g., weather, social media trends)
- Efficient model re-training strategies that balance computational cost with forecast accuracy
The competition results validate many of our architectural decisions, particularly the emphasis on computationally efficient models and robust evaluation frameworks. However, they also underscore the importance of building systems that can incorporate additional data sources and adapt to changing business conditions.
Future Directions
As forecasting systems continue to evolve, the focus increasingly shifts toward building robust, scalable architectures that can maintain competition-level accuracy while handling real-world complexities. The success of simpler models in the competition is relevant — adopting the latest NN and calling it a day is not likely to support repeatable success. Smart model decisions require nuance.
But scalable engineering, dynamic data capabilities, and managed feature engineering are all additional requirements for real-world success. At Syrup, our understanding of these needs allows us to provide advanced forecasting and merchandising capabilities that drive tangible financial results for some of the world’s biggest brands.
We extend our gratitude to Nicolas Vandeput for organizing the VN1 Competition and providing such a thorough analysis of the results. His insights into model performance and computational efficiency continue to resonate with real-world forecasting challenges and validate many of the architectural decisions made in production forecasting systems.
Recommended resources
See all resourcesMake Forecasting Your
Superpower
See how AI tailored to your unique business can deliver
insights that boost margin.
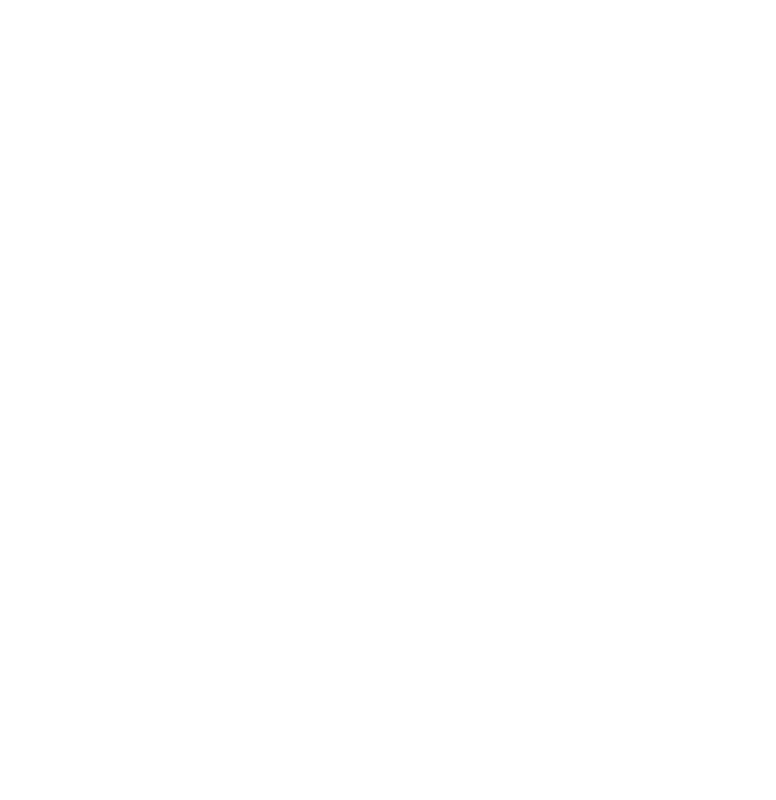