
Why Forecast Accuracy and Granularity Matter for Retail Allocators

Data is only as good as the next action it informs. With a forecast, it’s no different.
Forecasts inform actions that are the critical foundation for an allocator’s day-to-day work. But the business value of a given forecast is often explained using acronyms that mean little to anyone other than seasoned data scientists: MAPE, RMSE, MAE, etc.
While these error measurements have undeniable value, when engaging with allocators, we recommend re-framing the conversation to focus on the forecast’s ability to drive more effective allocation decisions.
For allocators navigating complex tradeoffs at the individual unit level (and down to the specific size, color, and store), that likely means a forecast that is granular, accurate, and reliable — that is, you believe it.
Ultimately, if your current forecast isn't helping you optimize allocation with greater precision, leading to stronger performance, it's falling short of its potential.
Data That Drives Action
There is value, of course, in tracking trends over time and comparisons between categories or forecasting models, but to really understand the business impact of a forecast from an allocator’s perspective, we need to link it back to the real world decisions the forecast supports.
Allocation decisions boil down to:
- How many units do I think we’re going to sell in this store? This is, practically, the forecast. The information feeding the decision needs to be granular to the level at which the decision is being made, and of course, believable.
- How much safety stock do I think we need? The optimal answer to this should be heavily influenced by confidence in the forecast. If an allocator doesn’t really trust the forecast, their strongest course of action is to hedge their bets with a safety stock bump.
You’re probably noticing that these required decisions hint at some important measurement needs related to accuracy — this is where the RMSE MAE acronym salad I mentioned earlier comes into play. I won’t dive into the nitty gritty of how these things are measured, but suffice to say that it involves some relatively complicated math and the output is usually not super comprehensible to the average person who is not a data scientist.
An alternative is using forecast “accuracy.” For example, if a forecast is 90% accurate, it matched the actual sales we saw for that SKU in that store for that week 90% of the time. This has the benefit of being more immediately understandable — but it requires several layers of ambiguation, moving us further away from “does this inform my next action.”
Through the lens of an allocator, evaluating a forecast's effectiveness goes beyond simply looking at overall accuracy metrics. You need to understand how well it equips you to take decisive action at the level where it matters most — the individual unit. If your forecast isn’t providing the clarity and precision needed to make these decisions with confidence and accuracy, it’s not serving its core purpose.
Let’s explore three features of a good allocations forecast.
The 3 Hallmarks of an Actionable Allocations Forecast
A truly effective allocations forecast goes beyond surface-level predictions. It possesses key characteristics that directly empower better decision-making and improved business outcomes.
1. Granularity that Matches Your Decisions
Imagine trying to decide how many size small blue shirts to send to your flagship store in San Francisco based solely on a total apparel forecast for the West Coast. It's a recipe for inefficiency. A useful forecast provides granularity that mirrors the specificity of your required actions — down to the size-store level.
Looking at forecast error at this granular level, such as the total units error for a specific SKU in a particular location, reveals the frequency with which forecast inaccuracies lead to tangible problems: stockouts due to under-allocation or excess inventory due to over-allocation. This detailed insight enables far more precise allocation decisions and can significantly reduce the need for large safety stock buffers.
2. Errors Within Acceptable Limits
While perfect prediction is unattainable, understanding and minimizing forecast errors is crucial. Common metrics like wMAPE, MAE, and RMSE provide statistical measures of forecast accuracy. However, the real value lies in understanding how these errors translate into business impact. A high wMAPE for an aggregate category might mask significant inaccuracies at the size-store level, leading to missed sales and markdowns.
Having safety stock above the forecast value is a key part of allocation. Ideally, error metrics should recognize this and acknowledge that revenue is only lost when the margin of error exceeds safety stock at an SKU-store level.
3. Direct Impact on Key Performance Indicators
Ultimately, a good forecast should directly contribute to your core goals: avoiding stockouts/overstocks, maximizing sell-through, and protecting margins. This is where the impact of allocation error becomes clear.
Even if the total quantity purchased is correct, inaccurate forecasts at the store level lead to misallocation. Sending too much of a particular size or style to a store where demand is low results in “trapped” stock, where we run down DC inventory and some stores stock out while inventory sits on the shelf elsewhere, and eventual markdowns. Conversely, under-allocating to high-demand locations leads to mid-season stockouts and lost sales opportunities.
As detailed in our resource on allocation error, this can also damage customer loyalty and brand perception when shoppers can't find what they're looking for.
A granular and accurate forecast minimizes these errors, ensuring the right product is in the right place at the right time, maximizing full-price sell-through and reducing the need for costly markdowns.
Now that you know what to look for, it’s probably helpful to think about the “so what?” question that often follows.
What is the Value of a “Better” Allocations Forecast?
Intuitively, a good allocations forecast — one that meets the requirements covered in the last section — provides value by improving allocation outcomes. But it’s less easy to say, for example, what the difference is between a forecast that is 70% accurate and one that is 80% accurate.
Observed correlations from McKinsey suggest that improvements in forecast accuracy can indeed have a significant impact: “improving forecasting accuracy by 10 to 20 percent translates into a potential 5 percent reduction in inventory costs and revenue increases of 2 to 3 percent.”
Different sources quote different figures, and the true impact will depend on factors like product type and existing operational efficiency. However, the directional relationship is strong: more accurate forecasts generally translate to a healthier bottom line.
Here's what we consistently observe at Syrup:
- Measurable Gains in KPIs: Our customers experience tangible improvements, including an average of 10% increase in full-price sell-through while simultaneously holding up to 30% less inventory. This optimized inventory management and increased sales efficiency directly contribute to margin improvements of around 5%.
- Empowered and Efficient Teams: Beyond the hard numbers, we consistently hear from our users that they gain significant efficiency and confidence in their day-to-day work. Trusting the forecast allows them to make quicker, more strategic decisions, reducing the time spent on reactive inventory management and freeing them to focus on higher-value activities.
- And Yes, Improved Aggregate Forecast Accuracy: While we don’t love using these metrics for proving value, they still have their place when comparing approaches and seeing trends. Our forecasting models consistently outperform pre-existing models by 20-50% at an aggregate level.
Our forecast outperforms some of the best forecasts available in head-to-head tests.
See the results
The Syrup Advantage: Forecasts Engineered for Actionable Insights Across Merchandising Needs
I’ve focused this piece on allocations and their underlying hyper-granular forecasts, but of course, allocations are just one component of a much larger planning, buying, and merchandising machine. And it’s not possible to serve all of these needs with one forecast. Indeed, in some cases, granular forecasts are not helpful!
At Syrup, we solve this by developing and providing an ensemble model approach, with collections of models tuned to meet needs, whether you’re introducing a brand new product, opening a new store, planning demand across an assortment, or yes, making weekly allocations to maximize sell-through and margin.
No matter what your next action is, we have you covered.
Recommended resources
See all resourcesRecommended resources
See all resourcesMake Forecasting Your
Superpower
See how AI tailored to your unique business can deliver
insights that boost margin.
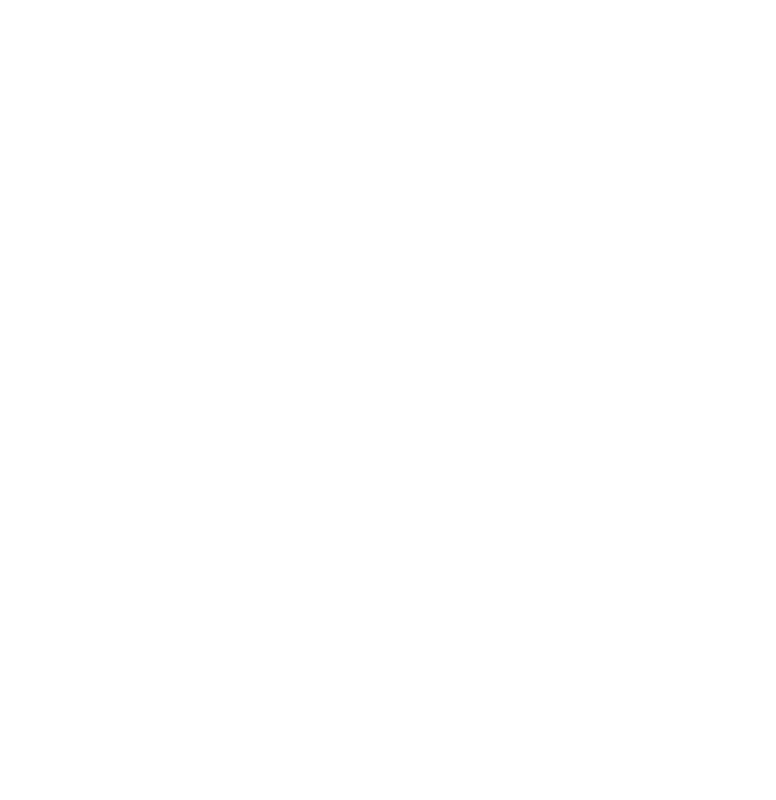